Analysis of the risk of road flooding
The challenge at hand is to use spatial data analysis techniques to assess the impact of coastal storm surges on road infrastructure. Specifically, the task is to identify road segments that are at risk of being submerged by a coastal surge.
The geometry of the road network data is derived from an open municipal data source that details the spatial configuration of the roads. In addition, a Digital Elevation Model (DEM) is available that provides accurate elevation measurements for the terrain, including potentially flood-prone areas.
Given the variable altitude of roads and their significant lengths—especially in coastal areas—it is crucial to accurately model how these factors interact with the known height of the surge, which serves as a parameter in our analysis. The objective is to develop a predictive model that can delineate the road portions susceptible to inundation by the surge, taking into account the complex interplay between road elevation, surge height, and the topographical nuances captured by the DEM.
This problem requires a deep understanding of geospatial data manipulation, elevation data analysis, and the ability to apply advanced data science techniques to predict and visualize the impact of environmental factors on road infrastructure.
Initial assessment of the complexity of the analysis
Spatial resolution of DEM Data
The Digital Elevation Model (DEM) provides elevation data with a relatively high accuracy of 30 meters per pixel. However, this resolution presents a challenge in accurately mapping DEM pixels to specific points along road geometries. The granularity of the DEM data may not perfectly match the finer details of road elevations, especially when considering the intricate and variable elevation profiles of coastal roads.
Road geometry representation
The road network data is presented as continuous lines (one line per road) rather than segmented sections. This representation lacks the granularity needed to accurately assess the impact of a coastal surge on specific road segments. The continuous line model does not easily account for the variable elevation and length of roads, making it difficult to determine which sections are at risk without further segmentation.
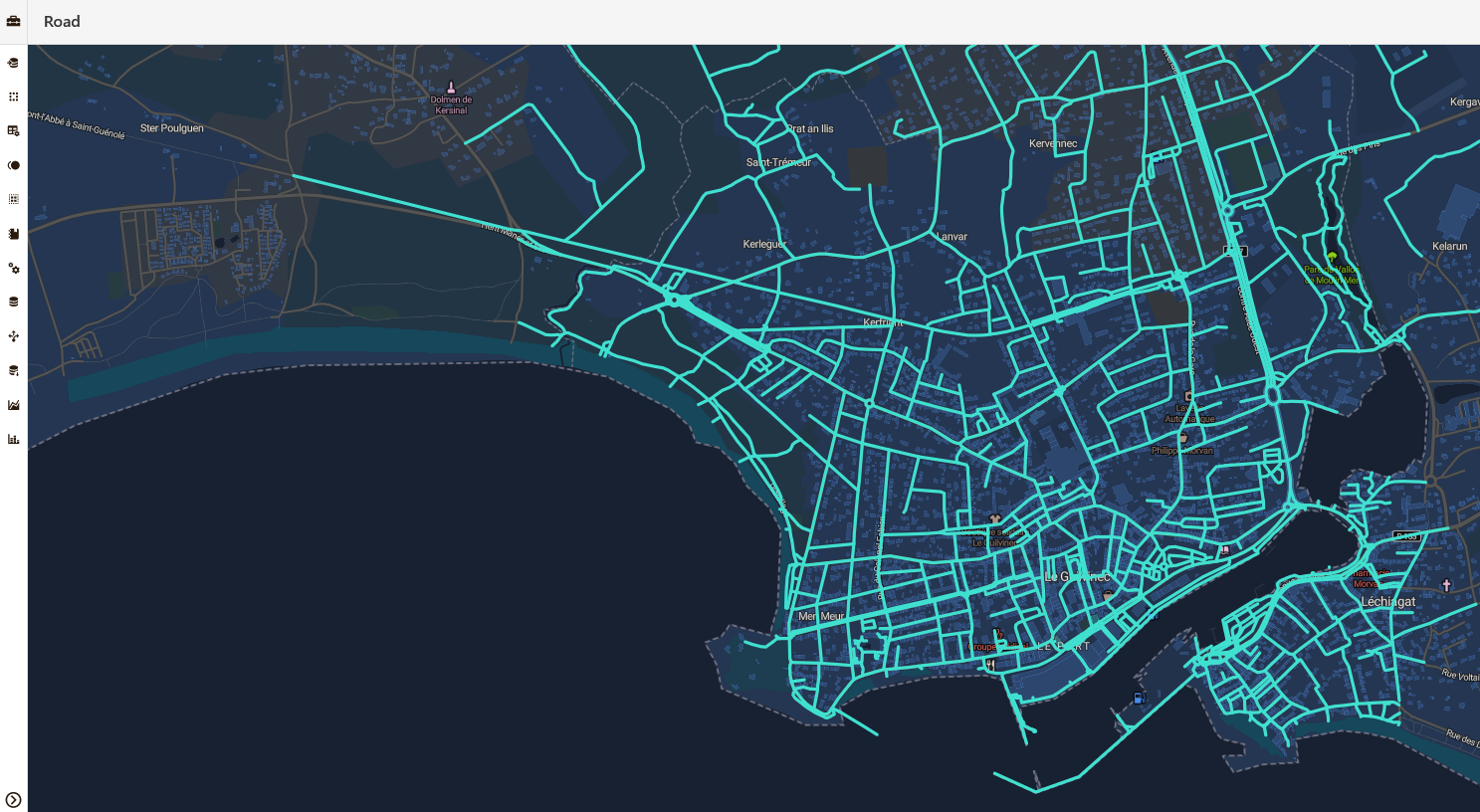
Computational efficiency and scalability
Calculating elevation for each point along the road would require significant computational resources, given the extensive lengths of coastal roads. This process involves intensive spatial analysis and elevation data extraction for numerous points, which could become prohibitively expensive and time-consuming, especially when scaling the analysis to cover large geographic areas. The need for a scalable solution that can efficiently process and analyze large amounts of geospatial and elevation data is paramount to overcoming this challenge.
Assessing road flooding risk with the TranzAI Platform
Using the H3 geospatial indexing system to create a grid overlay of the geographic area of interest provides several benefits.
Spatial indexing and resolution flexibility
The H3 grid system provides a flexible framework for defining spatial resolution. By calculating the average elevation of each cell, you can effectively manage the resolution trade-off between computational efficiency and accuracy of elevation data. This flexibility allows the grid size to be adjusted to balance detail and processing requirements.
Segmentation of road networks
Creating road segments by intersecting roads with H3 cells is a pragmatic and efficient way to segment continuous road lines. This method naturally divides the road network into manageable pieces, each associated with the average elevation of a particular cell. This segmentation allows for a more granular analysis of which road segments are at risk of flooding, overcoming the problem of having a single line per road.